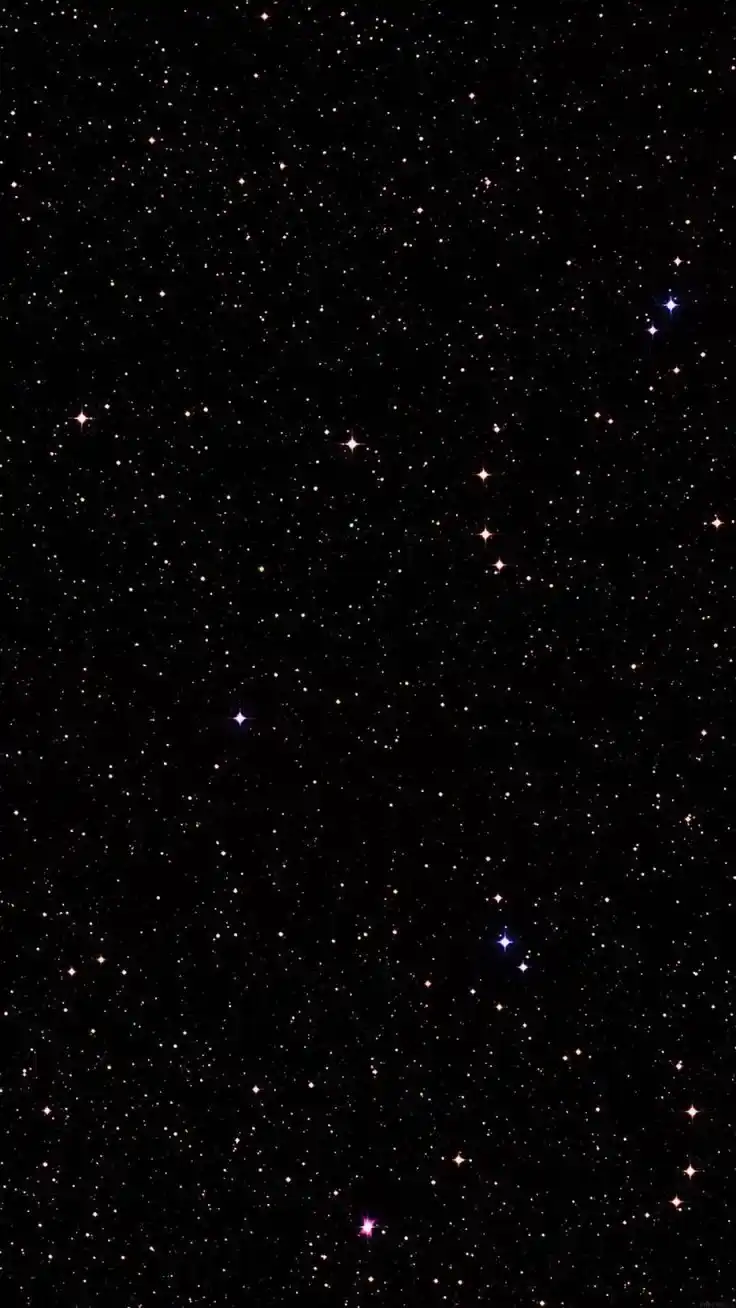
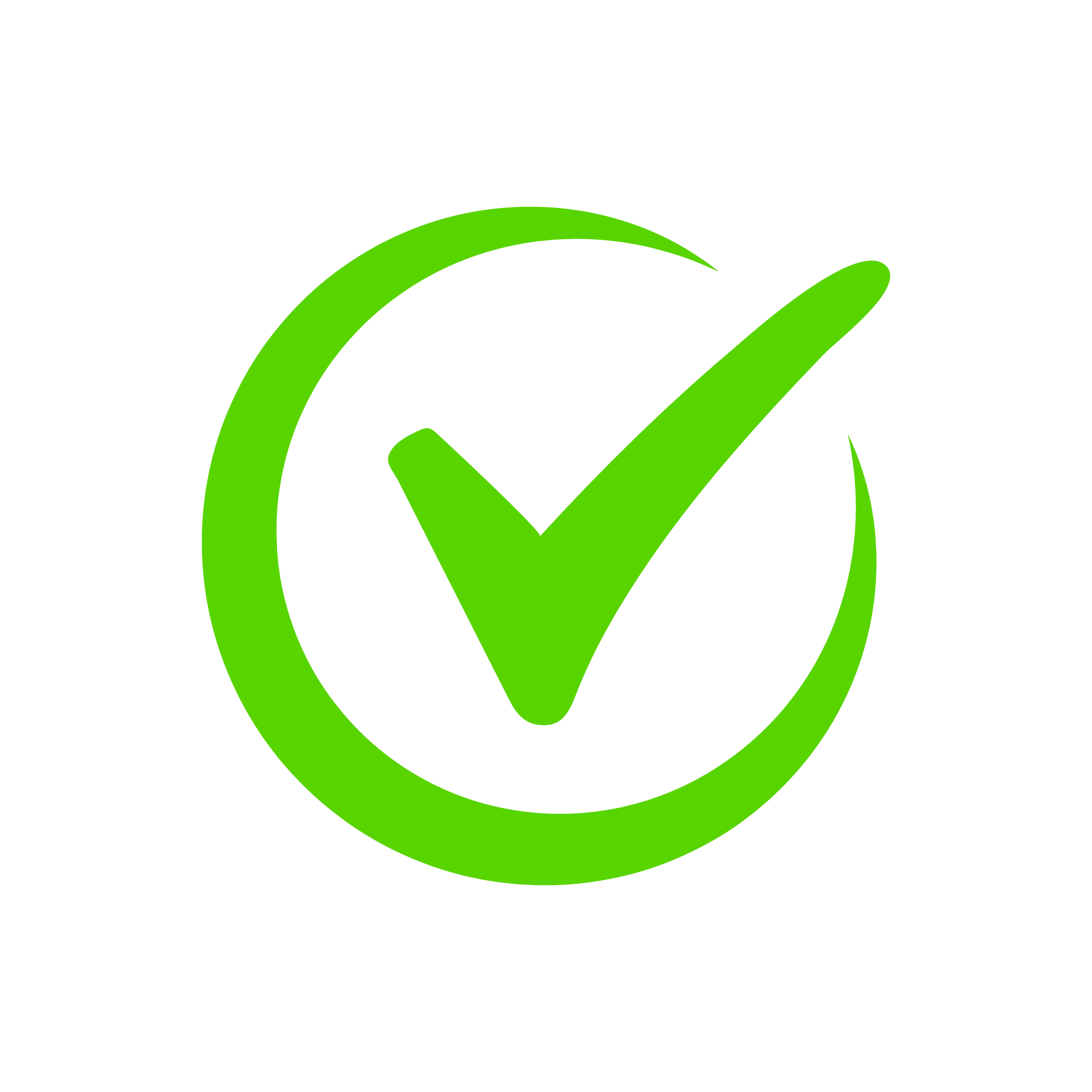
The source of the book
This book was brought from archive.org under a Creative Commons license, or the author or publishing house has agreed to publish the book. If you object to the publication of the book, please contact us.
The minimum description length principle
(0)
Author:
Peter GrünwaldNumber Of Downloads:
73
Number Of Reads:
9
Language:
English
File Size:
3.01 MB
Category:
Natural ScienceSection:
Pages:
50
Quality:
good
Views:
1194
Quate
Review
Save
Share
Book Description
The minimum description length (MDL) principle is a powerful method of inductive inference, the basis of statistical modeling, pattern recognition, and machine learning. It holds that the best explanation, given a limited set of observed data, is the one that permits the greatest compression of the data. MDL methods are particularly well-suited for dealing with model selection, prediction, and estimation problems in situations where the models under consideration can be arbitrarily complex, and overfitting the data is a serious concern. This extensive, step-by-step introduction to the MDL Principle provides a comprehensive reference (with an emphasis on conceptual issues) that is accessible to graduate students and researchers in statistics, pattern classification, machine learning, and data mining, to philosophers interested in the foundations of statistics, and to researchers in other applied sciences that involve model selection, including biology, econometrics, and experimental psychology. Part I provides a basic introduction to MDL and an overview of the concepts in statistics and information theory needed to understand MDL. Part II treats universal coding, the information-theoretic notion on which MDL is built, and part III gives a formal treatment of MDL theory as a theory of inductive inference based on universal coding. Part IV provides a comprehensive overview of the statistical theory of exponential families with an emphasis on their information-theoretic properties. The text includes a number of summaries, paragraphs offering the reader a "fast track" through the material, and boxes highlighting the most important concepts.
Peter Grünwald
Peter Grünwald heads the machine learning group at CWI in Amsterdam, the Netherlands. He is also full professor of Statistical Learning at the mathematical institute of Leiden University. Currently the President of the Association for Computational Learning, the organization running COLT, the world’s prime annual conference on machine learning theory, he was co-program chair of COLT in 2015 and also chaired UAI – another top ML conference – in 2010/2011. Apart from publishing at ML venues like NIPS, COLT and UAI, he also regularly contributes to statistics journals such as the Annals of Statistics. He is the author of the book The Minimum Description Length Principle, (MIT Press, 2007; see here for an up-to-date (2020), much shorter introduction), which has become the standard reference for the MDL approach to learning. In 2010 he was co-awarded the Van Dantzig prize, the highest Dutch award in statistics and operations research. He received NWO VIDI (2005), VICI (2010) and TOP-1 (2016) grants.
Read More
Read
Rate Now
1 Stars
2 Stars
3 Stars
4 Stars
5 Stars
Quotes
Top Rated
Latest
Quate
Be the first to leave a quote and earn 10 points
instead of 3
Other books by “Peter Grünwald”
Other books like The minimum description length principle
Comments
Be the first to leave a comment and earn 5 points
instead of 3